AI model enables earlier detection of diabetes through chest X-rays
A new artificial intelligence model that uses chest X-rays to predict risk for Type 2 diabetes could help physicians detect the disease earlier and prevent complications.
The multi-institutional study, conducted in collaboration with researchers from University of Illinois Chicago and Duly Health and Care and published in Nature Communications, finds that X-ray images collected during routine medical care can provide warning signs for diabetes, even in patients who don’t meet the guidelines for elevated risk.
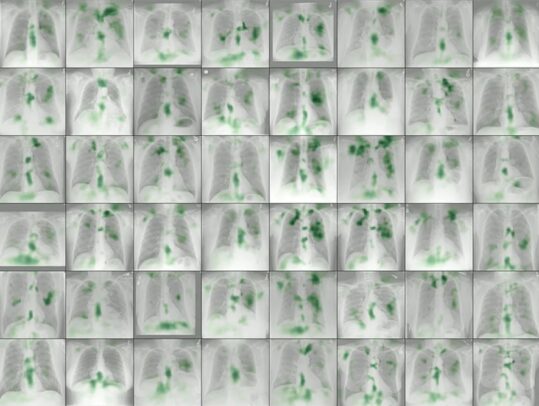
The team built a model that successfully flagged elevated diabetes risk, often years before patients were diagnosed with the disease, by applying the computational method known as deep learning to images and electronic health record data. Additional research found that the model made its predictions based on the location of fatty tissue.
“We do a lot of these exams for patients, and we know that there’s lots of data in these images that we just haven’t really figured out a way of extracting,” said Dr. Ayis Pyrros, adjunct professor at UIC, radiologist at Duly Health and Care and lead author of the study. “A tool like this can be helpful to identify these higher-risk patients without extra cost or effort.”
Current guidelines suggest screening patients for Type 2 diabetes when they are between 35 to 70 years old and considered overweight or obese according to their body mass index (BMI). However, many studies have shown that these guidelines can miss a significant number of cases, particularly when BMI is a less effective predictor of diabetes risk.
Simply screening everyone for diabetes would be costly and inefficient. But chest X-rays provide an opportunistic alternative — each year, millions of Americans receive these exams for chest pain, difficulty breathing, injury or before surgeries. While radiologists are not looking for diabetes when they assess these images, they become attached to a patient’s medical record and could be analyzed later for diabetes or other conditions.
“This is a way to use AI to pull out new data from tests typically used for other reasons,” said Dr. Brian Layden, professor of medicine and chief of the division of endocrinology, diabetes and metabolism at UIC and co-author of the study. “When doctors take a chest X-ray to see if a patient has pneumonia, they can also ask if there are other health issues that should be considered. That’s a really exciting and innovative idea.”
The AI model was trained on over 270,000 X-ray images from 160,000 patients, with deep learning determining the image features that best predicted a later diagnosis of diabetes. When the researchers applied the model to a separate group of nearly 10,000 patients, they found that their model predicted risk better than a simple model that didn’t use image data.
Within that population, the model found 1,300 patients at high risk of diabetes even though they did not have a formal diagnosis or measurement of high blood sugar at the time. Of those, nearly 150 also did not meet the age and BMI criteria for diabetes screening.
“It shows the opportunity to catch these patients that just don’t know they have diabetes,” Pyrros said. “It’s well known that sometimes patients have high blood pressure and high blood sugar, but just don’t really experience any symptoms for quite some time.”
On average, the chest X-ray warned of high diabetes risk three years before the patient eventually received a diagnosis. That advance notice could be incredibly valuable for catching the disease in its initial stages and starting treatment and lifestyle changes before serious complications arise.
“There are a lot of people with diabetes, but there are also a lot of people with pre-diabetes, and it’s important to not let them go undiagnosed in the early stages where damage is starting to build,” Layden said. “Any way to decrease that delay in diagnosis will save people from early complications and progression of disease.”
Because chest X-rays are not a common way to detect diabetes, the research team also used explainable AI techniques to determine how and why it made its determinations. The methods pointed to the location of fatty tissue as important for determining risk, a logic that aligns with recent medical findings that visceral fat in the upper body and abdomen is associated with Type 2 diabetes, insulin resistance, hypertension and other conditions.
The research team will now explore how to further validate the model and incorporate it into electronic health record systems, providing an alert to physicians to pursue the traditional blood sugar test for diabetes screening in patients flagged as high risk based on X-ray results.
They’re also exploring how chest X-rays can be used to diagnose other conditions, such as vascular disease, congestive heart failure and chronic obstructive pulmonary disease. The model’s output also provides a numerical risk score that could potentially help clinicians customize the treatment approach for diabetic patients.
“There are many patients with pre-diabetes in the U.S., nearly 100 million. Some of these patients will develop diabetes and some will not. We would like to see if the addition of the machine learning risk score to traditional screening can better predict which patients with pre-diabetes are most likely to develop diabetes,” said Dr. Bill Galanter, associate professor of medicine at UIC and co-author of the study.